Big data, the specter of AI, and the long-awaited switch to CSA have IT and Quality leaders asking: “what’s next?” On February 27th, 2024, three industry experts sat down to answer that question.
Panelists
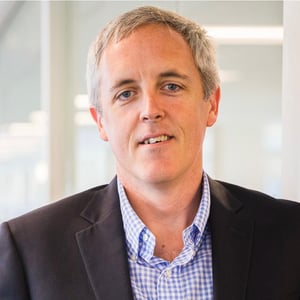
Xilio Therapeutics
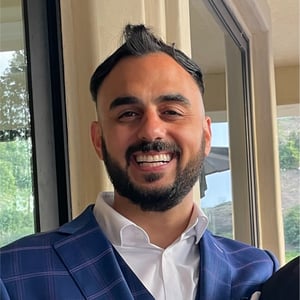
SpringWorks Therapeutics
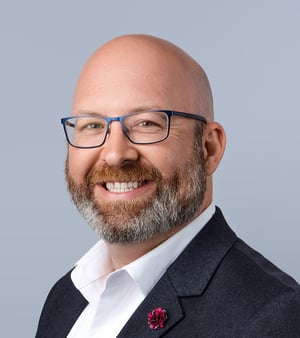
Sware
TABLE OF CONTENTS
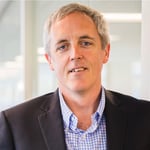
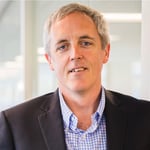
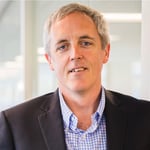
Nathan McBride
Senior Vice President, IT
Xilio Therapeutics
Nathan McBride
Senior Vice President, IT
Xilio Therapeutics
Nathan McBride
Senior Vice President, IT
Xilio Therapeutics
Disclaimer: Statements made by our panelists are their opinions and not affiliated with or reflective of their respective companies.
As the speed and connectivity of the life sciences industry evolves, the old way of performing computer system validation (CSV) – using pen and paper, or a hybrid digital-analog approach – isn’t just inefficient, but a liability.
As tech stacks grow larger and release updates more frequent, legacy models will soon fail to keep pace, leading to bad data, unaddressed risks, and significant material consequences.
Here are three things your life sciences company should be doing now to prepare for the industry’s future:
-
Focusing on data over AI
-
Switching from CSV to CSA
-
Strengthening collaboration between IT and Quality

Nathan McBride: “For most companies, what was once a normalized data generation curve is now a huge peak upswing. Knowing that this was coming, we started training everybody on how to think about using AI-supported functionality on top of these new platforms. Our staff started to figure out how to use these new functions to write a question on top of the data that they already have and use those questions to generate actionable information.”
Focus on Data Today and AI Tomorrow
As the volume and complexity of generated data increases, effective governance becomes paramount. Life sciences companies are facing a surge of valuable data as their systems modernize and mature. To action that data responsibly, they must establish robust data management frameworks, prioritizing data integrity, security, and accessibility.
Effective data management and governance requires specialized training and functional domain expertise. IT and Quality teams must possess specialized knowledge, including a nuanced understanding of data ingestion, storage, and extraction. Building effective warehousing, data lakes, and other data architectures is necessary to bridge the gap between real-world activity and the incorporation of actionable insights.
Ultimately, a company’s data management goal should be to define and leverage metadata to isolate and protect the top 1% of information: the “sovereign data” that is essential, actionable, and shared in the board room.
Today, there is a frenzy of discussion around the future role generative AI, but its full potential has yet to materialize. Tools like Microsoft Copilot are being used to speed up routine administrative processes. Companies are exploring the solution landscape to identify systems for highly specific purposes. Standard SaaS platforms like Asana, Monday, Smartsheet, and Box are all offering AI support, but the industry is far from the point of “agreement” on the best practices and best technologies to use.

Bryan Ennis: “We’re moving from a calculus to a statistics kind of world. In Quality, we’ve been very binary: it works, or it doesn’t. And now, as we look at these learning models, it’s hard to wrap your head around the nuanced confidence intervals based on automation. But what might’ve taken you eight or nine months to do as an organization can now be done in weekend.”
In the future, it is likely (or perhaps inevitable) that AI will be able to handle more complex and comprehensive tasks. But poor business process understanding, and data management today means inaccurate, AI-based results tomorrow, as the reliability of any AI-based system is entirely contingent on the quality of the addressed business processes and data it receives.
Barriers to AI Adoption
The biggest barriers to AI adoption, according to IDC’s October 2023 Global AI (Including GenAI) Buyer Sentiment, Adoption, and Business Value Survey are cost, lack of skilled staff, and lack of governance and risk management protocols. Concern about “data poisoning,” wherein bad data seeds and proliferates, abound, as do concerns about security, privacy, and job displacement. For companies that are adopting AI (approx. 45% of respondents), time and resources are being spent to “enforce rules, policies, and processes” to enforce responsible AI governance. However, according to the same survey, 87% feel that their organizations are “less than fully prepared to take advantage of GenAI capabilities in the next 24 months.”
Excerpt Source: Daitaku
Three Most Important Business Outcomes from GenAI
Q: Which of the following are the three most important business outcomes that your organization is trying to achieve from AI initiatives?
n=697
Source: IDCS’s Global AI (Including GenAI) Buyer Sentiment, Adoption, and Business Value Survey, October 2023
Excerpt Source: IBM

Nathan McBride: “In order to get to a point in time where you can use AI to generate good data, you need to have human beings in that loop. If you’re doing quality on development, you’ll end up doing quality on data. Companies that aren’t using metadata today will eventually realize that they’re sitting on top of a mountain of bad data. And the only way to mitigate that is to start now. Last year, in fact.”
Bryan Ennis: “With SDLC, we’re once again reentering a paradigm that places emphasis on quality. As we leverage more AI and automated capabilities in engineering and product management processes, we need to focus on data to properly assess product quality and how we govern it. As a Quality leader with an AI background, how do you think about this, in terms of prioritizing quality system design and the kind of controls that need to be in place?”
Aryaz Zomorodi: “I think people are hesitant to move towards AI because of one question: how do you validate something that is ever-changing? You need to have very well-versed individuals that understand how data is coming into this model, how statistical probabilities are being calculated. There’s an ideal reliability score,” but today, there’s no clear-cut way to get there. The regulations are not up to speed yet. As we all know, the paradigm of CSA was developed years ago, and we’re only now getting somewhere with it.”
Manage Risk by Adopting CSA
CSV as it exists today is no longer sufficient for providing timely and risk-assured software validation. The time has come to start talking seriously about CSA: computer system assurance, a risk-based paradigm that increases efficiency by replacing end-to-end “blanket” testing – a “test everything” approach – with risk-prioritized testing.
CSA is a risk-first paradigm that uses systematic, objective risk assessment to identify areas of risk and align them with appropriate levels of testing. Ultimately, this creates inspectable evidence that demonstrates compliance with a greater degree of specificity and much higher efficiency.
FDA Code 21 CFR Part 11 defines Electronic Records and Electronic Signatures requirements used by pharmaceutical or medical device manufacturers when they submit to the US FDA to show compliance with cGMP practices. The regulations “set forth the criteria under which the agency considers electronic records, electronic signatures, and handwritten signatures executed to electronic records to be trustworthy, reliable, and generally equivalent to paper records and handwritten signatures executed on paper.” This advisory publication forms the basis of the computer system assurance paradigm, which most companies have been slow to implement due to time and resource constraints.
Excerpt Source: Perficient
The increasing volume of software applications (and their regular releases) that need to be validated can overwhelm IT and Quality teams that are already being asked to do more with less. Life sciences teams are increasingly relying on their vendors to demonstrate the quality of their products and identify what capabilities present impact and risk to OOTB and configured business processes. When vendors can clearly demonstrate and articulate that functionality does not present a direct impact to patient safety, product quality, data integrity, and/or security, life sciences companies leverage CSA risk-assessment to reduce unnecessary testing activities and keep their focus on business operations.

Aryaz Zomorodi: “To regulators, the purpose of CSA is to leverage vendor documentation in an intelligent way. Right off the bat, the imperative is to stop trying to test and retest where your vendor’s already done it, and instead, use critical thinking to identify risk within your process (and overall intended use) and the testing effort would be commensurate the risk. That’s something auditors/inspectors would like to see: and yes, that you have a robust change management process. You have audit trails, audit logs, all those things, which help support not needing to do endless rounds of testing associated with something that had very minimal risk.”
Implementing CSA can take several forms, but the utilization of Systematic Risk Assessments (SRA) is an effective route to take. SRA is used to routinely identify and score risks, eschewing blanket testing to focus on high-liability elements.
SRA can be broken down into several broad phases:
-
Concept: Existing and newly added SaaS systems are risk-assessed to determine system and business impact. Risk-based decisions are subsequently identified and developed.
-
Project: Functional risk assessments are performed to identify impact on patient safety, production quality, and data integrity. Controls are defined.
-
Operation: Controls are verified and implemented. Risks are continuously monitored, and parameters are responsively adjusted.
-
Retire & Archive: Risk-based decisions are factored when retiring or archiving systems.
Advanced workflow automation is a powerful, if not essential way to execute these steps. An automated validation process can perform SRA and use metadata to operationalize SOPs that IT and Quality leaders can use to increase reliability, transparency, and audit-readiness across a variety of use cases and scenarios in clinical development, manufacturing, and commercial operations. It also helps establish a consistent, scalable change management process that allows teams to quickly flag and remediate contingencies in the event of a high-impact error.
Today, compliance stewards frequently receive tested data packages and validate by hand, printing and signing documentation. This process, the cornerstone of traditional CSV, is not only slow and inefficient, but risk prone. Intelligent workflow automation systems based on calibrated rulesets can both accelerate validation time and build risk assurance directly into key validation workflows.
In the broader software ecosystem, workflow management is already a major disruptor. Powerful tools allow companies across industries to create automated workflows tailored specifically to their needs. This capability is emerging in the life sciences validation space, where modern workflow engines are being developed to make end-to-end, paperless validation dramatically faster, more secure, risk-proofed, and transparent.
Quality Risk Management Process
Form a United Front
Effective preparation for technology innovation, as well as making the switch from CSV to CSA, depends entirely on strong collaboration between IT and Quality teams. Both teams should act as one seamless unit to ensure that an efficient, effective, risk-managed process is carried out consistently. Often, the relationship between IT and Quality manifests as a negotiation – focused on time, cost, and outcomes – when it should play out collaboratively.
Ultimately, IT is responsible for innovating and executing, and Quality for ensuring risk is effectively managed. The advent of AI-supported tools and shift to CSA may excite or discourage members of either team, but both teams will need to play an equal role in realizing their potential upsides. IT leaders should be well versed in Quality’s perspective, and Quality leaders must understand the driving principles behind IT.
Hiring a team with cross-functional and multidisciplinary skills is a good first step, but rounded talent can be difficult to come by. Integrating the skills and knowledge of third-party industry experts is frequently more time- and cost-efficient than trying to assemble every piece in-house, especially when major initiatives – such as process conversion or AI preparation and integration – are on the line.
Nathan McBride: “There’s no more building on your own. A long time ago, IT and Quality came to an agreement: we’re going to build these things, and we need you to help us qualify them. Then the arrival of cloud computing changed everything. So the question now is: how deep do we go in terms of layers? My argument to my Quality folks is always: let’s stop right here. Let’s go as low as we must, but no lower than that. And depending on who I get to partner with, we can either go way down deep or we can stay right there.”
Aryaz Zomorodi: “First, I’m a huge advocate of IT and Quality working well together. The relationship must be strong because we need to support each other in this very fast-paced environment. I do agree that you want to come to a threshold where you say, ‘we can’t go any lower because you’re going to expend too much effort for little value add.’ I haven’t been put in this situation yet, so thankfully I haven’t had that. But I do think that collaboration, having a documented approach on how deep to go and how best to meet regulations, is critical.”
Prepare for the Future with Sware
The future of life sciences validation and compliance is not so far away. Already, we are seeing life sciences companies rush to integrate AI where possible, while at the same time striving to manage risk with fewer human and material resources available. Facing an influx of new SaaS system releases, increasingly complex web of integrations, and shrinking talent pool, many companies are at a loss regarding how to move forward.
Sware is here to help. As the industry’s leading expert on life sciences software validation, Sware helps IT and Quality teams navigate the challenges, bottlenecks, and successes that come with a rapidly evolving and constantly transforming industry. Sware’s Res_Q platform, backed by world-class functional domain expertise, automates, unifies, and accelerates life sciences CSV and CSA. Res_Q reduces validation time by up to 80%, associated costs by up to 60%, and provides superior risk assurance every step of the way.
To learn more about Sware, Res_Q, and the future of life sciences validation, request a demo.
Featured Resources
Learn how Nuvolo is leveraging a combination of Sware's Res_Q Platform and expert resources to deliver a fully GxP compliant offering to the life sciences industry, enabling Nuvolo and their customers to meet business goals.
Read the case study